Hanqing Tang
Eval:
Unveiling Pain
Pain perception is a subjective experience, leading to inconsistencies in assessment and management. This thesis delves into the intricacies of this problem and proposes the development of a wearable device for objective pain assessment. By detecting physiological parameters, particularly Electrocardiography (ECG), the device aims to provide an accurate and unbiased measure of pain intensity. This study endeavors to validate the device's accuracy while exploring potential gender, race, and age-based differences in pain perception. The overarching goal is to address the gap in pain evaluation, ultimately enhancing the quality of pain care for patients across diverse medical and research settings.
Pain: A Multifaceted Experience
Image
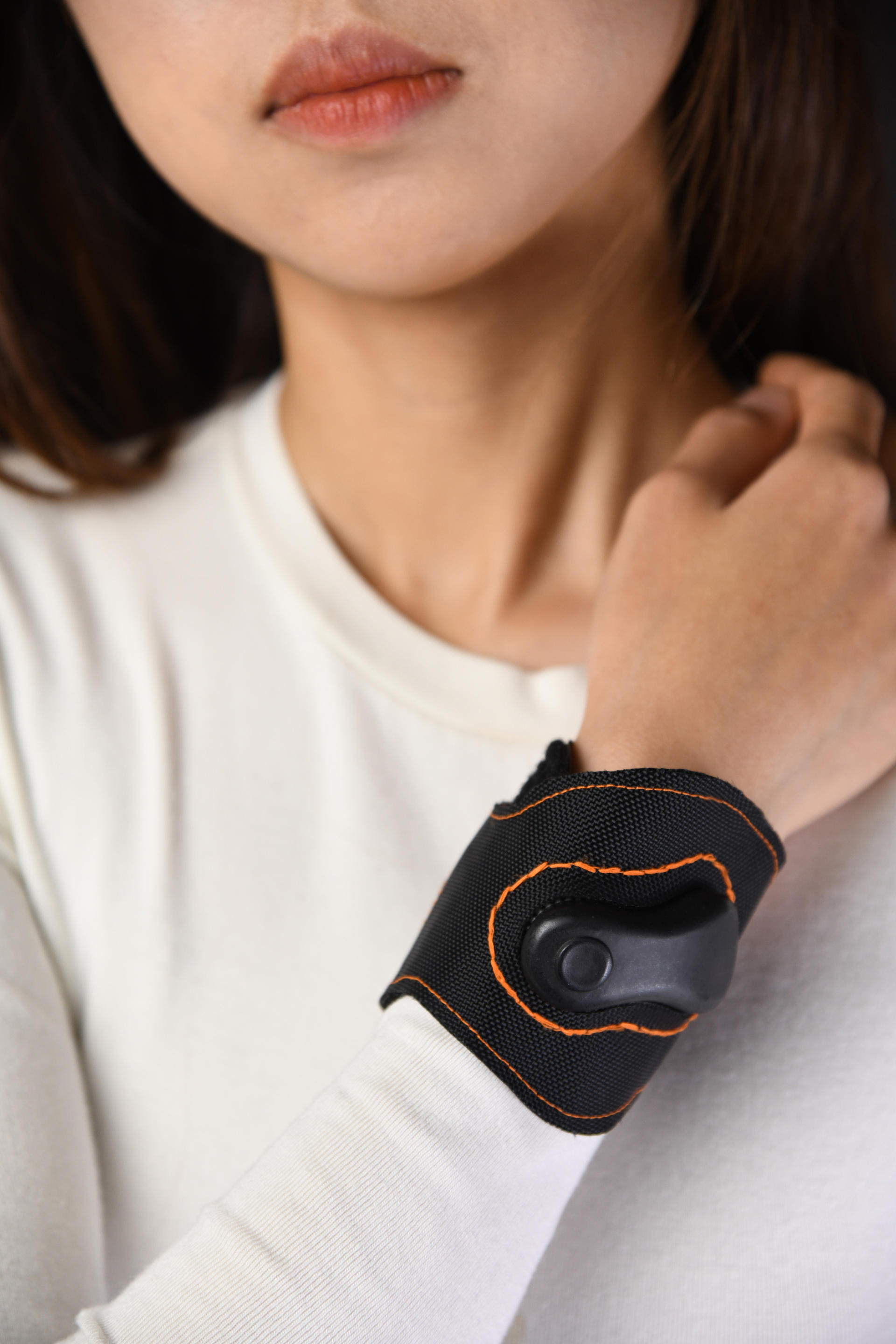
Pain is a highly individual sensation that involves a complex interplay of sensory, emotional, cognitive, and social factors, often associated with actual or potential tissue damage
Image
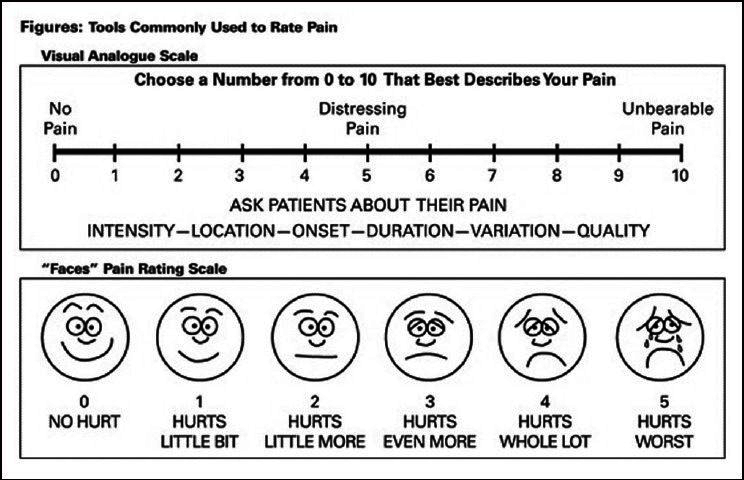
In the clinical and research settings, the traditional pain estimation methods such as the Visual Analog Scale or Numeric Rating Scale, where the patient rates their pain on a scale from 0 to 10 is subjective
Understanding Pain Perception Beyond Self-Report
Image
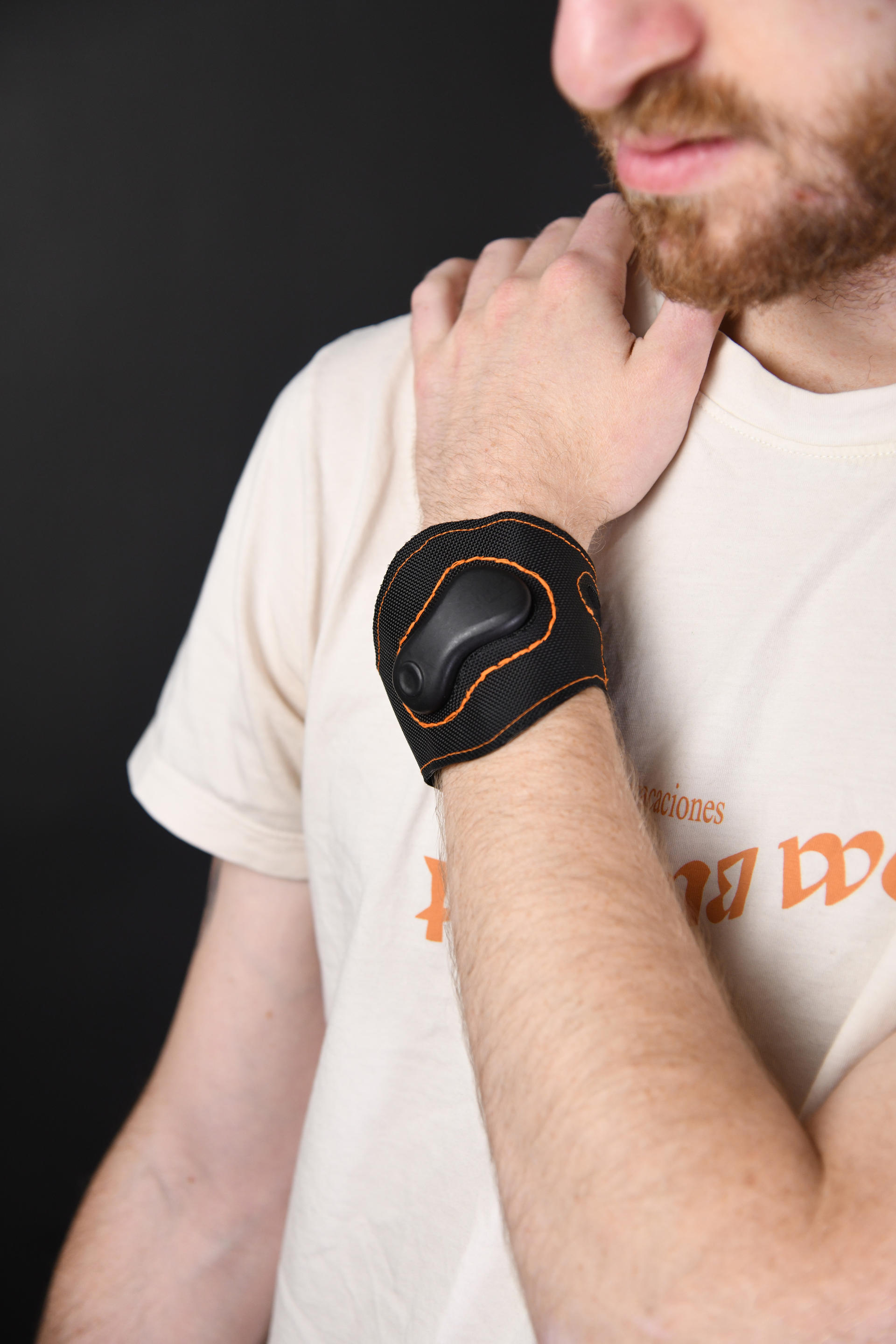
Self-report of pain levels may differ from personal pain experience, as it is a controlled and goal-directed response to pain, which may be affected by reporting bias and differences in memory and language abilities. Pain perception is also influenced by various factors, such as genetics, gender, age, and previous experiences.
Empowering Precision Pain Care
Image
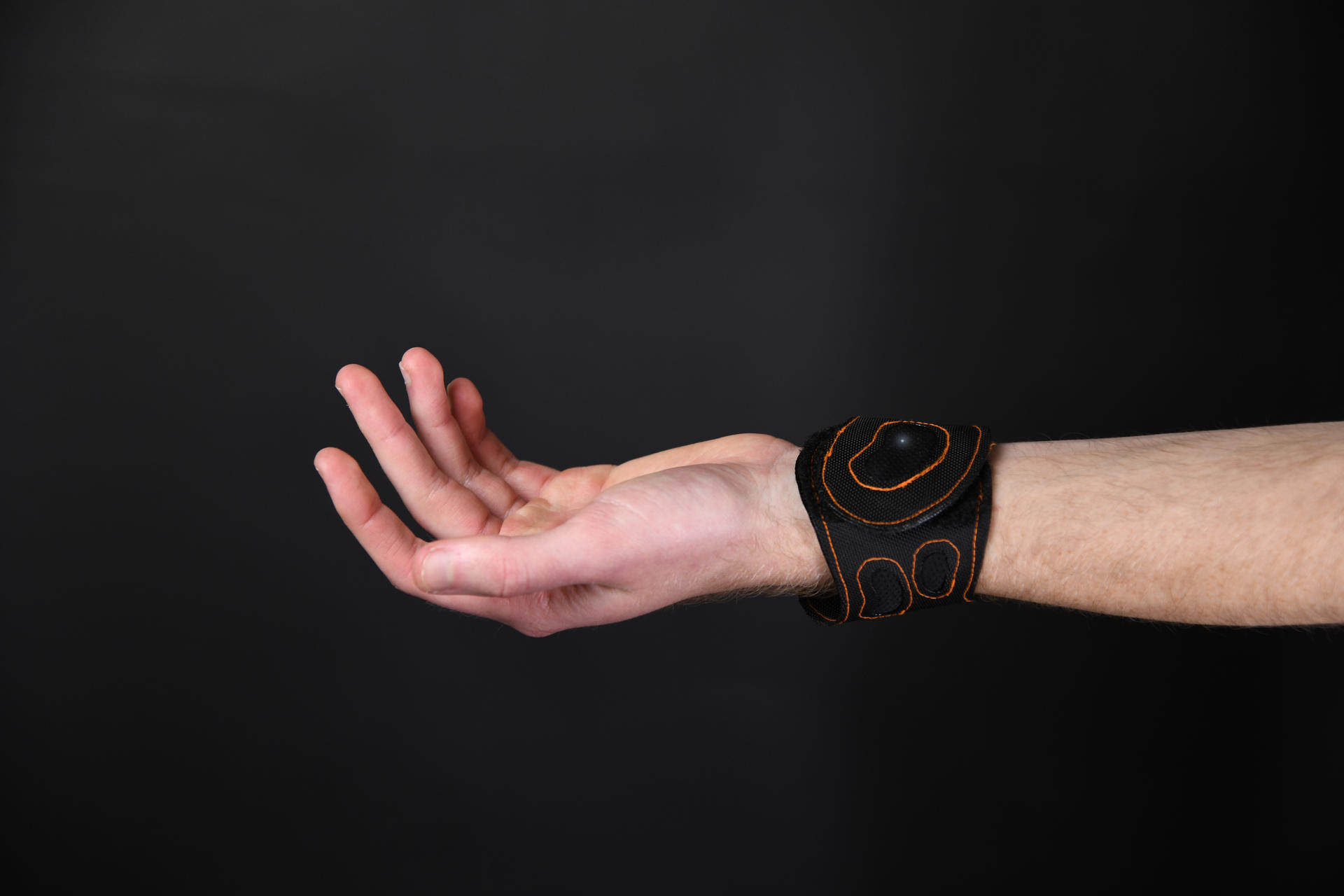
A wearable pain measuring device that tells patients’ pain level based on evident physiological parameters will let physicians perceive patients' pain experience accurately and offer appropriate healthcare.
Utilizing Physiological Parameters as Evidence
Image
Physiological measures can offer more reliable and objective pain information. Heart rate variability (HRV), measured through electrocardiograms (ECGs) from wearable devices or monitors, provides an autonomic regulation index of heart rate and serves as a dependable source for acute pain monitoring.
Machine Learning for Pain Intensity Classification
Recent studies demonstrate that machine learning methods, trained using the BioVid pain database, can accurately classify heart rate and acute pain intensity. This research applies machine learning models, trained through the BioVid database to determine evidence-based pain intensity using physiological measures.
Pain in Healthcare
Image
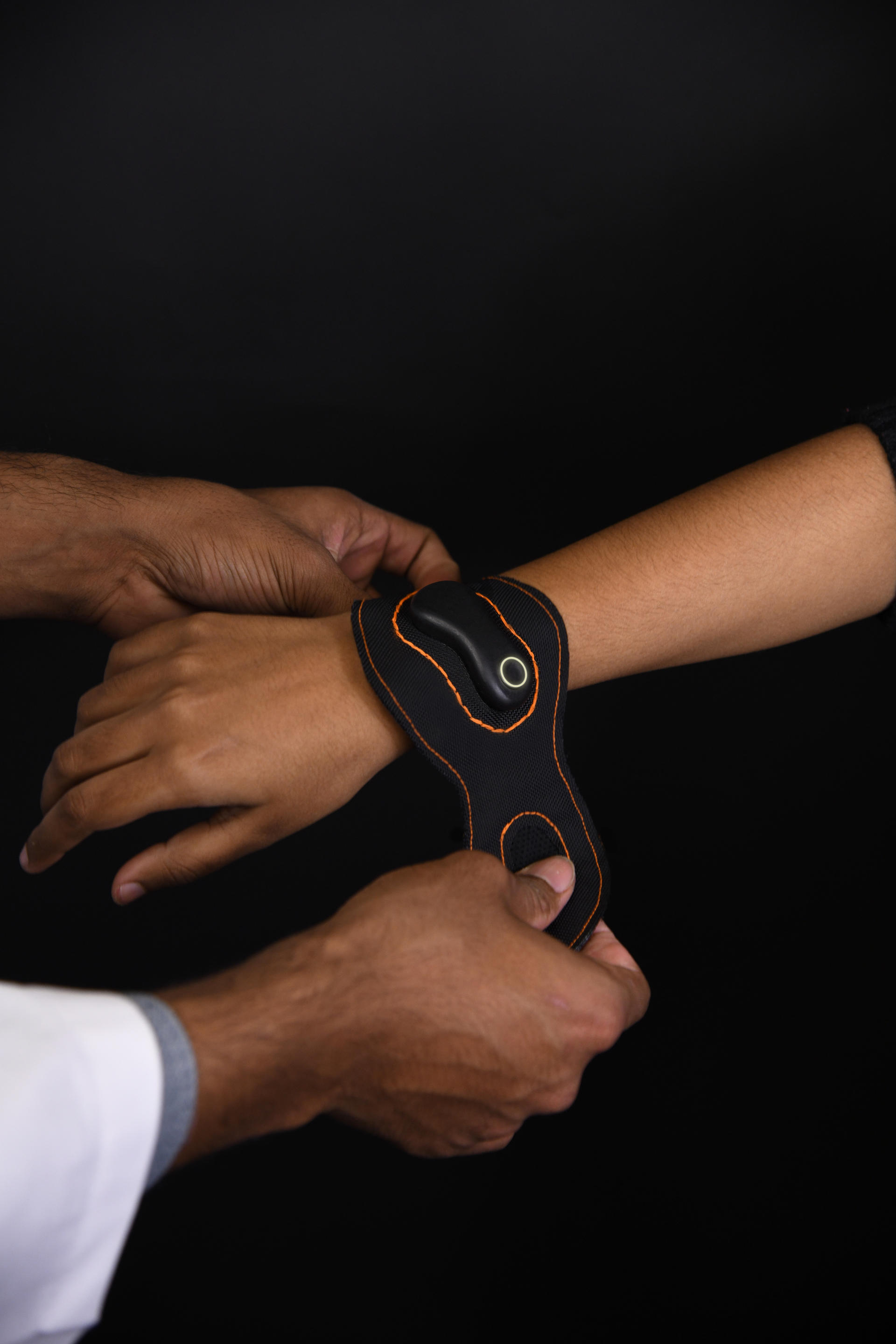
This device is intended to detect acute pain in the waiting room when patients are seeking medical assistance. The initial assessment will be conducted by a reception desk nurse who will record the patient's pain level and other relevant information upon entering the waiting room.
Unleashing the Healing Power
Image
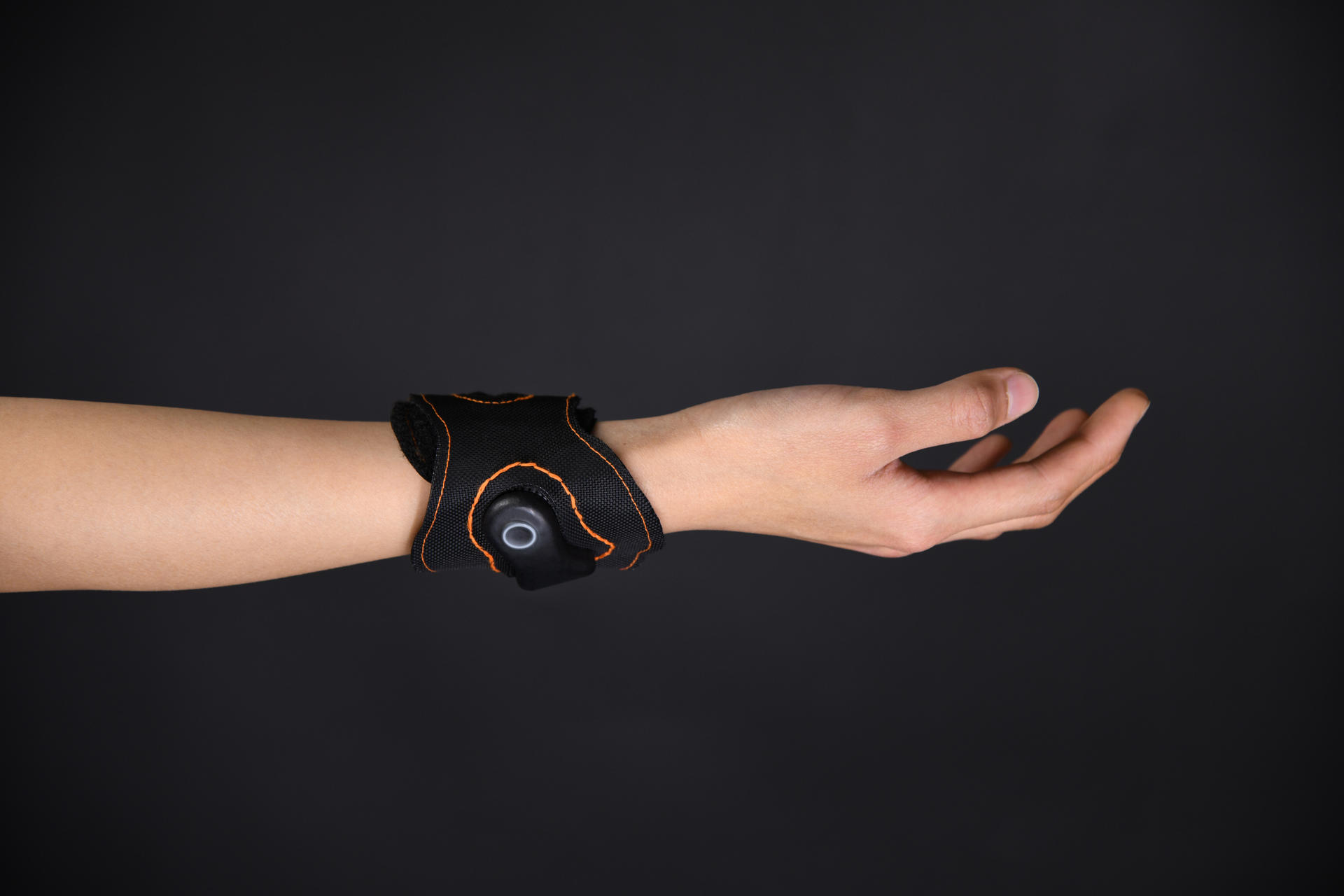
The wearable pain measuring device exhibits potential for improving pain management in diverse medical and research settings, including pain level monitoring during surgical procedures and tracking pain levels in individuals with chronic pain conditions.
Prosperity for All
Image
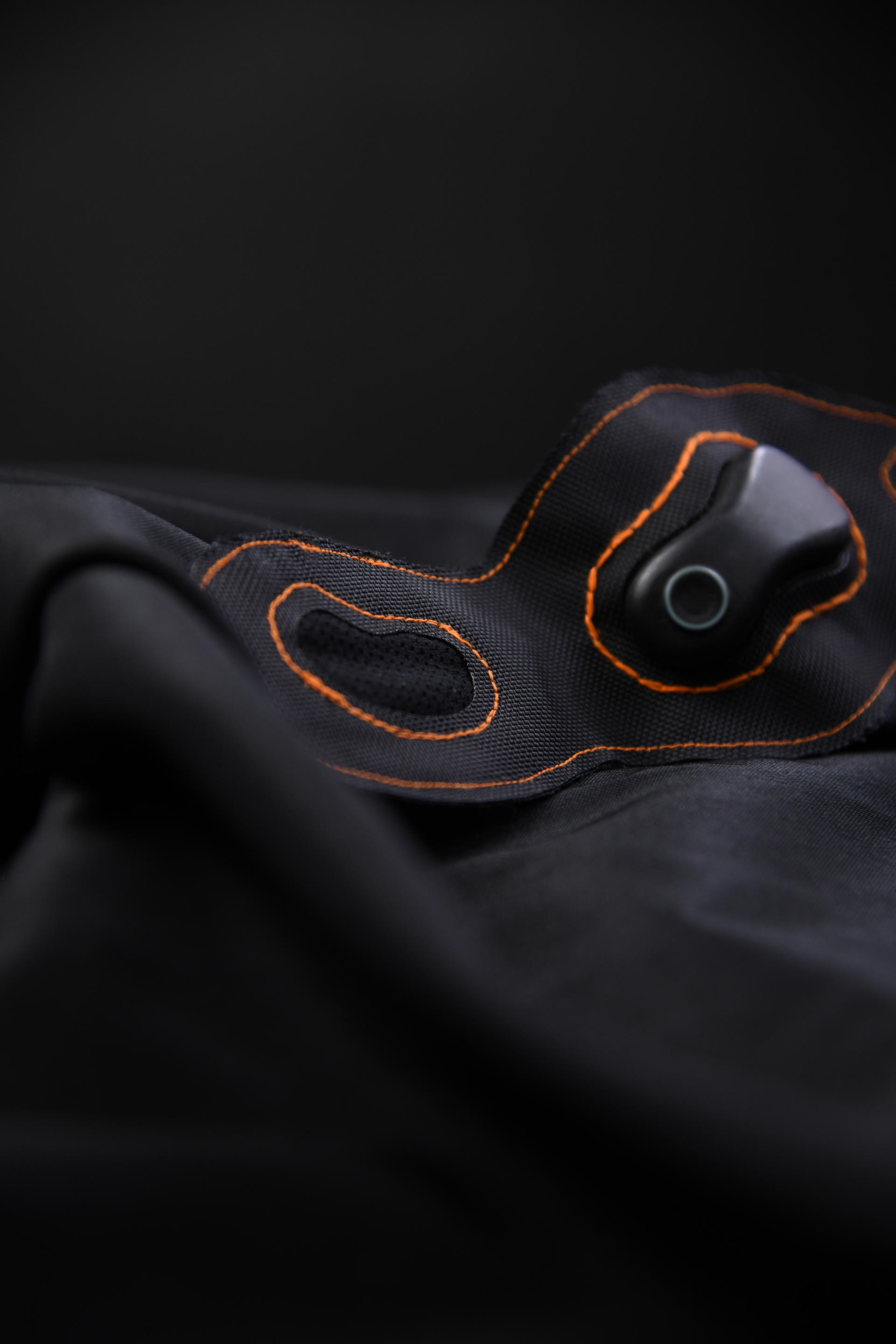
Ensuring equitable access to care and treatment for individuals of all races, ages, and genders necessitates confronting bias in pain evaluation. By offering an objective measure of pain, this wearable device holds immense promise in mitigating disparities across these dimensions, fostering fairer and more inclusive pain assessment and management practices.